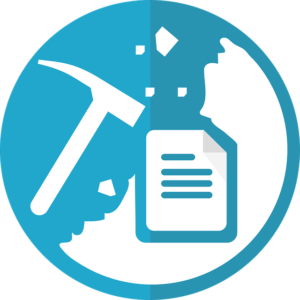
Archaeological grey literature reports were primarily a response to the explosion of archaeological work from the 1970s (e.g. Thomas 1991) which generated a backlog which quickly outstripped the capacity of archaeologists, funders, and publishers to create traditional outputs, and it became accepted that the vast majority of fieldwork undertaken would never be published in any form other than as a client report or summary format. This in turn (and especially in academic circles) frequently raised concerns over the quality of the reports, as well as their accessibility: indeed, Cunliffe suggested that some reports were barely worth the paper they were printed on (cited in Ford 2010, 827). Elsewhere, it was argued that the schematisation of reports could make it easier to hide shortcomings and lead to lower standards (e.g. Andersson et al. 2010, 23). On the other hand, it was increasingly recognised that such reports had become the essential building blocks for archaeological knowledge to the extent that labelling them ‘grey’ was something of a misnomer (e.g. Evans 2015, sec 5), and the majority of archaeological interventions across Europe were being carried out within the framework of development-led archaeology rather than through the much smaller number of more traditional research excavations (e.g. Beck 2022, 3).
In the face of the challenges of manually extracting data from grey literature reports discussed in the previous post, in particular the friction costs in time and energy to reuse the data, archaeologists have been increasingly turning to automated or semi-automated methods of data extraction. Initial efforts focused on automating the creation of ‘what where when’ metadata for cataloguing purposes. For instance, the Archaeology Data Service used Named Entity Recognition techniques to extract the subject (‘what’), location (‘where’), temporal information (‘when’), grid references (‘where’ again), alongside the title, authorship, and date of the report in their Archaeotools project (Richards et al. 2011, 44-45) which ultimately lies behind the faceted search tools used today in Archsearch.
More recent projects have sought to go beyond this automated cataloguing emphasis and mine the grey literature to answer specifically archaeological research questions. For example, Alex Brandsen has recently employed machine learning (e.g. Brandsen et al. 2021) in conjunction with a dataset of 65,000 Dutch grey literature reports to look at early medieval cremation practices in Holland (Brandsen and Lippok 2021). The system was able to add 23 previously unknown sites to the corpus, representing a 30% increase in known cases. Interestingly, the study was based on a very low level of retrieval precision – using various terms for cremation plus a date range as the search criteria, a total of 2541 documents were returned of which 2446 were determined to be irrelevant, a precision level of 2.1%. The majority of irrelevant results were caused by incorrectly identified time periods, while other errors were caused by the inclusion of lists of abbreviations and time periods, bibliographies etc. which never relate to a described excavated cremation (Brandsen and Lippok 2021, 4). Of course, determining whether a returned report was relevant or not was a determination requiring a human review, so not an insignificant task in this case.
A rather different emphasis which takes us one stage further is found in another new study, by Gertjan Plets, Pim Huijnen, and David van Oeveren (2021), who suggest that text mining can also be used as a means of studying the history and evolution of archaeological knowledge creation rather than simply extracting data associated with contemporary archaeological practice (Plets et al. 2021, 290). Their study focussed on Belgium and employed a broad range of sources, including grey literature reports. Their objective was to trace the development of theoretical trends through the texts, the impact of organizational circumstances on knowledge production, and the extent to which nationalist framings of the past are evident. I’m only going to refer here to their examination of organisational circumstances on knowledge creation, as it directly relates to grey literature. For example, they compared the similarity of archaeological reports with preceding years, essentially measuring the levels of self-plagiarism. They argue that the texts produced by commercial organisations are much more similar to each other than reports by universities and governmental agencies indicating that reports relied on a greater degree of boiler templates (Plets et al. 2021, 296 and figure 4). They also looked at lexical density and observe a marked difference with texts produced by commercial organisations. For much of the period from 1950- 2000 the lexical density remains steady and high, with an index fluctuating around 0.8 which linguists characterise as very dense and connected to analytical writing rich in analytical words. From 2000 onwards, as archaeology becomes increasingly part of the planning process, lexical density decreases and by 2010 texts are half as dense as compared to 2000. While texts by universities and government agencies still fall within the range of what would be called expository texts, reports by commercial companies fall below this threshold and are within the range of general prose or spoken language (Plets et al. 2021, 296-7 and figure 6). The decrease in complex vocabulary and the widespread use of boiler templating is striking, and they argue indicates a dramatic fall in quality.
Alarmingly, Plets and colleagues question whether such reports have sufficient methodological and theoretical depth to sustain their use in large-scale synthetic interpretative studies (Plets et al. 2021, 297). Set alongside this criticism, as already seen in the context of grey data, the work undertaken by Mike Fulford and Neil Holbrook evaluating contemporary professional practice (2018, 216) highlighted a number of shortcomings in the collection and presentation of information within grey literature reports. Further, we can see that the manual extraction of data from grey literature is time-consuming, costly, and subject to considerable variability, and while semi-automated methods have been applied, to date at least these still require a considerable degree of human intervention and validation although doubtless this will change as systems develop and become more refined. All this suggests that anxieties regarding the reliability of grey literature expressed from the earliest days of its production may be resurrected, but perhaps this time with more empirical data to reinforce those concerns.
All of which speaks to a need to evaluate the quality of grey literature reports properly, rather than just take them at face value as something to be mined for data in the assumption that their quantity will outweigh any issues of variability and unreliability. This makes it all the more important to be able to assess the journey between site and report. For instance, the typical drawing in a report resolves a range of doubts and uncertainties which are inherent in archaeological field drawings and this can be seen by setting the final drawing alongside the corresponding archived scan of the original field drawing by way of comparison (assuming it is available). Their differences highlight areas of uncertainty and ambiguity which were somehow resolved through decisions taken during the post-excavation process. Data changes as it travels between field and final drawing, for reasons that are rarely explained. How much of this is just ‘tidying up’, how much of it is changed interpretation, how much of this is error in transcription? We simply don’t know.
What this also illustrates is another key issue with semi-automated approaches to grey literature. They all focus on the text, whereas archaeology is a highly visual practice and archaeological reports typically contain a substantial amount of illustrative information alongside the text (Huggett, forthcoming). The problem, however, is that compared to text it is much more difficult to automatically or semi-automatically extract information from visual imagery. Indeed, the most common approach is to derive textual descriptions of images, which seems a rather circular approach and a poor substitute for what the viewer would see. An alternative approach is to use neural networks which can be trained to recognise objects and categorise images. This is not without its problems however – for instance, algorithms do not look at an image the way humans do, so it is often difficult to be sure exactly what the system is ‘seeing’ when it categorises images and it may be using backgrounds or giving other elements unexpectedly false prominence. To compound the problem there is also a general lack of interpretability of these systems making it difficult to understand how a classification has been arrived at (e.g. Huggett 2021, 427ff). There is also the problem that most neural networks are trained on photographs, and usually photographs of modern items at that, so although we might use such a system to distinguish between photographs and illustrations within an archaeological report, they would not be able to categorise and classify the diagrams in any meaningful way. We would need to train a system up from scratch to acquire expertise in archaeological illustration, which is not a simple thing to attempt. Interestingly, Plets and colleagues follow up their textual analysis by suggesting that future work might incorporate neural networks for the automated classification of images (2021, 301), so we might expect there to be examples coming forward in the next few years.
So where does this leave us? Piecing together the various fragments of evidence in the absence of a coherent evaluation of grey literature, there are clearly questions to be asked. These range across accessibility (in the sense of ease of access to the data within), reliability (the data frictions in tracing the journey from field to report, the variability of content, and key data absences), and quality (the effects of standardisation of reporting templates and boilerplate text, and the limitations of presentation and expression, for instance). Add to this the customary concerns regarding grey literature and the practices surrounding its creation and the picture surrounding archaeological grey literature is replete with uncertainty. Seeking to overcome this inherent uncertainty and variability through large-scale ‘big data’ style analysis of large numbers of reports in the belief that it will even out their biases and shortcomings seems a forlorn hope, and it is certainly unwise to assume that automated retrieval methods will somehow overcome any deficiencies. And yet the significance of the body of grey literature, its size, its scope, and its growth year on year, makes it all the more important that attempts are made to find effective ways of working with the material, and the examples offered by Brandsen and Plets et al., for example, provide a useful starting point for developing new approaches to grey literature and its associated data.
[This post is part of a presentation given to GRASCA, the Graduate School in Contract Archaeology at Linnaeus University on 7th June 2022. Thanks to Cornelius Holtorf and colleagues for their invitation and generous hospitality]
References
Andersson, C., Lagerlöf, A. and Skyllberg, E. (2010) ‘Assessing and Measuring: On Quality in Development-led Archaeology’, Current Swedish Archaeology, 18(1), pp. 11–28. https://doi.org/10.37718/CSA.2010.01.
Beck, A.S. (2022) ‘An Overlooked Frontier? Scenes from Development-led Archaeology Today’, Norwegian Archaeological Review, pp. 1–4. doi:https://doi.org/10.1080/00293652.2021.2010124.
Brandsen, A., Veberne, S., Lambers, K. and Wansleeben, M. (2021) ‘Can BERT Dig It? Named Entity Recognition for Information Retrieval in the Archaeology Domain’, Journal on Computing and Cultural Heritage [Preprint]. https://doi.org/10.1145/3497842.
Brandsen, A. and Lippok, F. (2021) ‘A burning question – Using an intelligent grey literature search engine to change our views on early medieval burial practices in the Netherlands’, Journal of Archaeological Science, 133, p. 105456. https://doi.org/10.1016/j.jas.2021.105456.
Evans, T. (2015) ‘A Reassessment of Archaeological Grey Literature: semantics and paradoxes’, Internet Archaeology, 40. https://doi.org/10.11141/ia.40.6.
Ford, M. (2010) ‘Archaeology: Hidden treasure’, Nature, 464 (7290), pp. 826–827. https://doi.org/10.1038/464826a.
Fulford, M. and Holbrook, N. (2018) ‘Relevant Beyond the Roman Period: Approaches to the Investigation, Analysis and Dissemination of Archaeological Investigations of the Rural Settlements and Landscapes of Roman Britain’, Archaeological Journal, 175(2), pp. 214–230. https://doi.org/10.1080/00665983.2017.1412093.
Huggett, J. (2021) ‘Algorithmic Agency and Autonomy in Archaeological Practice’, Open Archaeology, 7(1), pp. 417–434. https://doi.org/10.1515/opar-2020-0136.
Huggett, J. (forthcoming) ‘Extending Discourse Analysis in Archaeology: A Multimodal Approach’, in Gonzales-Perez, C., Martin-Rodilla, P. and Pereira-Fariña, M. (eds.) Discourse and Argumentation in Archaeology: Conceptual and Computational Approaches. Cham: Springer.
Plets, G., Huijnen, P. and van Oeveren, D. (2021) ‘Excavating Archaeological Texts: Applying Digital Humanities to the Study of Archaeological Thought and Banal Nationalism’, Journal of Field Archaeology, 46(5), pp. 289–302. https://doi.org/10.1080/00934690.2021.1899889.
Richards, J.D. Jeffrey, S., Waller, S., Ciravegna, F., Chapman, S. and Zhang, Z. (2011) ‘The Archaeology Data Service and the Archaeotools Project: Faceted Classification and Natural Language Processing’, in E.C. Kansa, S.W. Kansa, and E. Watrall (eds) Archaeology 2.0: New approaches to communication and collaboration. Los Angeles: Cotsen Institute of Archaeology Press, pp. 31–56. Available at: https://escholarship.org/uc/item/1r6137tb.
Thomas, R. (1991) ‘Drowning in data?’, Antiquity, 65(249), pp. 822–828. https://doi.org/10.1017/S0003598X00080546.