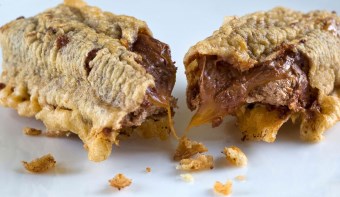
I’ve borrowed the idea of ‘deep-fried data’ from the title of a presentation by Maciej Cegłowski to the Collections as Data conference at the Library of Congress last month. As an archaeologist living and working in Scotland for 26 years, the idea of deep-fried data spoke to me, not least of course because of Scotland’s culinary reputation for deep-frying anything and everything. Deep-fried Mars bars, deep-fried Crème eggs, deep-fried butter balls in Irn Bru batter, deep-fried pizza, deep-fried steak pies, and so it goes on (see some more not entirely serious examples).
Hardened arteries aside, what does deep-fried data mean, and how is this relevant to the archaeological situation? In fact, you don’t have to look too hard to see that cooking is often used as a metaphor for our relationship with and use of data.
Famously, Geoffrey Bowker wrote
“Raw data is both an oxymoron and a bad idea; to the contrary, data should be cooked with care.” (2005, 184).
This underlines that data do not exist in and of themselves; data are not ‘out there’, waiting to be discovered by the archaeologist. If anything, data are waiting to be created. Bowker’s image of ‘raw’ data is aligned with Levi-Strauss’s use of ‘raw’ as natural, untouched, and ‘cooked’ as the result of cultural processes (Bowker 2013, 168). Consequently he emphasises the cultural nature of data, such that it is situated, contingent, and incomplete, and as archaeologists, we are all-too aware (or should be) that data are theory-laden, created by specific people, under specific conditions, for specific purposes.
So archaeological data come already deep-fried – they are not untouched, pristine, but arrive as a consequence of pre-depositional and taphonomic changes and are further determined by our ability to recognise, recover, and record what we (choose to) see.
To take this further, Cegłowski’s deep-fried data are prepared for use within big data analysis or machine learning, which he likens to a deep-fat fryer – it can be used to cook pretty much anything (as we’ve seen). However, he warns:
“These techniques are effective, but the fact that the same generic approach works across a wide range of domains should make you suspicious about how much insight it’s adding”.
He goes on to ask:
“So what’s your data being fried in? These algorithms train on large collections that you know nothing about. Sites like Google operate on a scale hundreds of times bigger than anything in the humanities. Any irregularities in that training data end up infused into in the classifier.”
In this scenario, the training data used for machine learning is effectively the cooking oil, as John Naughton observes, – if it is contaminated by error, selectivity or bias, so too will be the patterns learned by the software (Naughton 2016). If this is a problem for classic applications of big data in commercial and business contexts, typically employing the data exhaust we leave behind from our browsing or shopping habits, etc., this is all the more true in the context of archaeological (big) data analysis.
One of the major challenges in archaeological data is that errors, selectivity and bias are almost impossible to quantify, difficult to allow for, and – often – overlooked. After all, the data are the data – they are messy, incomplete, but what choice do we have? We have to make do with what we have; the entities represented by those data are all too often gone so cannot be revisited. Welcome to the world of archaeological data.
Like all good analogies, the idea of deep-fried archaeological data breaks down if pushed too far. Deep-fried Mars bars are evidently not good for your health – just how bad seems open to debate although alarmist reports in newspapers that they increased the risk of a stroke were untrue. But that’s not to say that deep-fried data is bad for our archaeological health – quite the reverse. Not to recognise that our data are deep-fried is where the danger lies: we are always working with deep-fried data. It might be thought that there are exceptions to this: that data captured by instruments are ‘raw’, for instance, but this is a dangerous assumption – the choice of element to measure, the type of data to capture, is still down to the human agent, and hence is never as objective as we might like to think.
It’s often said of deep-fried Mars bars and their ilk that the fact that you can do it doesn’t mean you should. This is equally true of the uses to which we put our data – just because we can do some kind of analysis or create some sort of model, doesn’t mean that it is a good idea, especially when you consider that those data have been thoroughly deep-fried beforehand, quite probably in some cooking oil of uncertain pedigree.
References
Bowker, G. 2005 Memory Practices in the Sciences (Cambridge, MA: MIT Press).
Bowker, G. 2013 ‘Data Flakes: An Afterword to “Raw Data” Is an Oxymoron’, in L. Gitelman (ed.) “Raw Data” Is an Oxymoron (Cambridge, MA: MIT Press), pp. 167-171.
Cegłowski, M. 2016 ‘Deep-Fried Data’, http://idlewords.com/talks/deep_fried_data.htm
Naughton, J. 2016 ‘Machine learning: why we mustn’t be slaves to the algorithm’, The Observer, 16th October 2016 https://www.theguardian.com/commentisfree/2016/oct/16/slaves-to-algorithm-machine-learning-hidden-bias