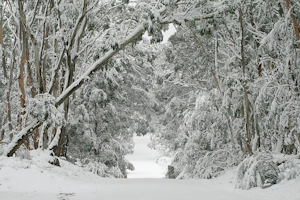
With Google’s introduction of ‘AI Overviews’ beginning to replace its traditional search engine, Apple launching its ‘Apple Intelligence’ system embedded in its latest variants of iOS, Adobe incorporating an AI Photo Editor in Photoshop, and so on, it’s fair to say that artificial intelligence – in the form of generative AI, at least – is infiltrating many of the digital tools and resources we are accustomed to rely upon. While many uncritically embrace such developments, others are asking whether such developments are desirable or even useful. Indeed, John Naughton (2023) suggests that we are currently in the euphoric stage of AI development and adoption which he predicts will soon be followed by a period of profit-taking before the AI bubble bursts.
In many ways, we’ve been here before. Haigh (2023, 35) describes AI as “… born in hype, and its story is usually told as a series of cycles of fervent enthusiasm followed by bitter disappointment”, and similarly,
The field of artificial intelligence has followed a pattern of boom and bust ever since its establishment in the 1950s. … AI is charged with hubris, grandiosity, claiming too much, reaching for the stars. Brought down to earth with a bump it had to slowly rebuild. The cycle gives us what were subsequently called ‘AI winters’, during which funding, and therefore research, froze. It is an internal, autochthonous historiography, part of the received culture of AI. It is both self-critical (we promised too much) and self-bolstering (but look at how ambitious our aims are). It blames both ancestors and outsiders. (Agar 2020, 291)
We’ve previously seen an AI bubble based around rule-based expert systems burst in the 1980s, leading to a 20-year slump in research and funding (Haigh 2024, 22). Archaeology experienced the same bubble – by the mid-1980s, a number of archaeological researchers (including myself!) were working on the development of expert systems though within a few years most activity had ceased, largely due to the limitations of the systems, problems formalising the knowledge base, and over-optimistic assessment of the tools (as critiqued in Huggett 1985; Huggett and Baker 1985, for instance) although it’s fair to say that not all agreed with this perspective.
The resurgence of AI in recent years has much to do with a significant paradigm shift away from the rule-based deductive approaches of expert systems to the inductive approaches of machine learning based on large quantities of data. Alongside the highly publicised flurry of commercial activities and applications, archaeology is also experiencing a resurgence in artificial intelligence applications, largely associated with feature extraction and identification, whether of sites from remotely sensed data or classification of artefacts according to types. Just as in the commercial world, we are seeing what at times seems like near-breathless enthusiasm in their adoption and use. For example, in their literature study of papers concerning AI and remote sensing in archaeology, Sobotkova et al. (2024, 7) found 63% of papers published mentioned no challenges or limitations at all, while the vast majority, whether or not they encountered difficulties, reported successful outcomes with only 6% reporting partial or complete failure. Even allowing for the difficulty and unattractiveness of publishing negative outcomes (Sobotkova et al. 2024, 7; Huggett 2018), this is a remarkable and improbably positive picture.
Reassuringly, therefore, we are beginning to see the appearance of more considered archaeological criticism, just as we did in the 1980s. For instance, Tenzer et al. (2024) discuss some of the ethical aspects of the introduction of artificial intelligence methods in archaeology, emphasising that “… as useful as the technology seems to be, it comes with a human and environmental cost.” The more extensive critique provided by Sobotkova et al. points to shortcomings associated with the archaeological application of machine learning approaches to satellite and LiDAR imagery and they “… offer a cautionary tale about the challenges, limitations, and demands of ML applied to archaeological prospection” (2024, 3). One of the key problems identified by Sobotkova et al. (2024, 3) and others (e.g. Huggett 2022, 285; Tenzer et al. 2024) surrounds the lack of transparency associated with the application of such tools – the lack of knowledge provided around the content and quality of training data, the tuning and use of pre-trained networks, and the nature of failures as well as successes. Both Sobotkova et al. (2024) and Tenzer et al. (2024) suggest a number of approaches to resolving the problems they identify but ultimately, they depend on an overall transparent approach which is largely lacking at present, making evaluation and assessment of the models and their results very difficult. As a consequence, our use of current artificial intelligence tools seems more than likely to suffer from a variety of biases ranging across initial research design, data collection, data analysis, and subsequent interpretation and publication (Fanelli 2012, 892), which we are currently unable to identify, quantify, and thereby correct.
Furthermore, although Tenzer et al. (2024) identify an environmental cost to the use of such tools, the point is not developed further. Previously, Richardson (2022) and Morgan (2022) have warned of the environmental costs of digital methodologies in archaeology but the problems are considerably enlarged with the adoption of AI. For instance, Luccioni et al. estimate that the development of their large language model cost a total of 50.5 metric tonnes of CO2-eq covering the manufacture of its physical servers and GPUs, the electrical power used in its training, and its associated network infrastructure, before considering the cost of deployment of its API (Luccioni et al. 2023, 5-7). By way of comparison, they estimate that OpenAI’s GPT-3 model used over 500 metric tonnes of CO2-eq in its training alone (2023, 10), while noting that commercial organisations are not transparent about such costs. At the same time, Li et al. (2023, 1) estimate GPT-3 also required 700,000 litres of fresh water during its training, while the global AI demand for water may be around half the UK’s total water withdrawal by 2027. The resource implications of these systems can be considerable, therefore, but this largely goes unaccounted for. Of course, the equivalent archaeological costs will be smaller than these, but nevertheless they remain largely hidden and much will be invisibly inherited through the use of pre-trained networks and development work undertaken elsewhere.
Given the human and environmental challenges of AI, an obvious question is whether it is all worthwhile. Its proponents would clearly argue that it is, pointing to time and effort saved in extracting information from vast tracts of data, but is this wholly justifiable? Others are more questioning, wondering whether the ends truly justify the means. For instance, as Molly White (2024) has observed,
… the reality is that you can’t build a hundred-billion-dollar industry around a technology that’s kind of useful, mostly in mundane ways, and that boasts perhaps small increases in productivity if and only if the people who use it fully understand its limitations. And you certainly can’t justify the kind of exploitation, extraction, and environmental cost that the industry has been mostly getting away with, in part because people have believed their lofty promises of someday changing the world.
Archaeology is certainly not a hundred-billion-dollar industry and seems unlikely to be about to change the world, but the kinds of questions Wright asks are just as applicable to archaeology as elsewhere, especially as it is becoming increasinly apparent that, despite the quantities of data consumed in their training, these new systems are most successful on relatively small, restricted, well-defined problems – just as the old ones were. Is this sufficient to warrant the underlying costs incurred?
References
Agar, J. (2020). What is science for? The Lighthill report on artificial intelligence reinterpreted. The British Journal for the History of Science, 53(3), 289–310. https://doi.org/10.1017/S0007087420000230
Fanelli, D. (2012). ‘Negative results are disappearing from most disciplines and countries’, Scientometrics, 90, 891-904. https://dx.doi.org/10.1007/s11192-011-0494-7
Haigh, T. (2023). There Was No ‘First AI Winter’. Communications of the ACM, 66(12), 35–39. https://doi.org/10.1145/3625833
Haigh, T. (2024). How the AI Boom Went Bust. Communications of the ACM, 67(2), 22–26. https://doi.org/10.1145/3634901
Huggett, J. (1985). Expert Systems in Archaeology. In M. A. Cooper & J. D. Richards (Eds.), Current Issues in Archaeological Computing, pp. 123–142. British Archaeological Reports. https://introspectivedigitalarchaeology.com/wp-content/uploads/2022/07/Huggett-Expert-Systems-in-Archaeology.pdf
Huggett, J., & Baker, K. G. (1985). The Computerised Archaeologist—The Development of Expert Systems. Science and Archaeology, 27, 3–12. https://introspectivedigitalarchaeology.com/wp-content/uploads/2022/07/Huggett-and-Baker-The-Computerised-Archaeologist-The-Development-of-Expert-Systems.pdf
Huggett, J. (2018). Is there a digital File Drawer problem? Introspective Digital Archaeology (16 April 2018) https://introspectivedigitalarchaeology.com/2018/04/16/is-there-a-digital-file-drawer-problem/
Huggett, J. (2022). Archaeological Practice and Digital Automation. In E. Watrall & L. Goldstein (Eds.), Digital Heritage and Archaeology in Practice: Data, Ethics, and Professionalism, pp. 275–304. University Press of Florida. https://doi.org/10.5744/florida/9780813069302.003.0013
Li, P., Yang, J., Islam, M.A., & Ren, S. (2023). Making AI Less ‘Thirsty’: Uncovering and Addressing the Secret Water Footprint of AI Models. arXiv. https://doi.org/10.48550/arXiv.2304.03271
Luccioni, A.S., Viguier, S., and Ligozat, A.-L. (2023). Estimating the Carbon Footprint of BLOOM, a 176B Parameter Language Model, Journal of Machine Learning Research, 24(253):1−15. https://www.jmlr.org/papers/v24/23-0069.html
Morgan, C. (2022). Current Digital Archaeology. Annual Review of Anthropology, 51(1), 213–231. https://doi.org/10.1146/annurev-anthro-041320-114101
Naughton, J. (2024). From boom to burst, the AI bubble is only heading in one direction, The Guardian (13 April 2024) https://www.theguardian.com/commentisfree/2024/apr/13/from-boom-to-burst-the-ai-bubble-is-only-heading-in-one-direction
Richardson, L.-J. (2022). The Dark Side of Digital Heritage: Ethics and Sustainability in Digital Practice. In: Garstki, K. (ed). Critical Archaeology in the Digital Age: Proceedings of the 12th IEMA Visiting Scholar’s Conference, pp. 201–210. Los Angeles: UCLA. https://escholarship.org/uc/item/0vh9t9jq#page=216
Sobotkova, A., Kristensen-McLachlan, R. D., Mallon, O., & Ross, S. A. (2024). Validating predictions of burial mounds with field data: The promise and reality of machine learning. Journal of Documentation (ahead-of-print). https://doi.org/10.1108/JD-05-2022-0096
Tenzer, M., Pistilli, G., Bransden, A., & Shenfield, A. (2024). Debating AI in Archaeology: Applications, implications, and ethical considerations. Internet Archaeology, 67. https://doi.org/10.11141/ia.67.8
White, M. 2024 ‘AI isn’t useless. But is it worth it?’, Citation Needed (17 April 2024) https://www.citationneeded.news/ai-isnt-useless/