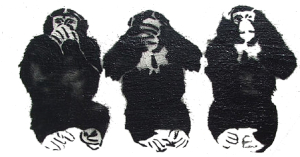
My employer has decided to send all those of us involved in recruitment and promotion on Unconscious Bias training, in recognition that unconscious bias may affect our decisions in one way or another. Unconscious bias in our dealings with others may be triggered by both visible and invisible characteristics, including gender, age, skin colour, sexual orientation, (dis)ability, accent, education, class, professional group etc.. That started me thinking – what about unconscious bias in relation to digital archaeology?
‘Unconscious bias’ isn’t a term commonly encountered within archaeology, although Sara Perry and others have written compellingly about online sexism and abuse experienced in academia and archaeology (Perry 2014, Perry et al 2015, for example). ‘Bias’, on the other hand, is rather more frequently referred to, especially in the context of our relationship to data. Most of us are aware, for instance, that as archaeologists we bring a host of preconceptions, assumptions, as well as cultural, gender and other biases to bear on our interpretations, and recognising this, seek means to reduce if not avoid it altogether. Nevertheless, there may still be bias in the sites we select, the data we collect, and the interpretations we place upon them. But what happens when the digital intervenes?
In some respects, nothing changes. For example, Charlotte Beck and George Jones (1989) discussed bias in artefact analysis, identifying three dimensions of bias: the explicitness of attribute and class definitions being applied; differences in perception among analysts; and changes in an analyst’s perception over time (1989, 245). At first glance, simply introducing the digital into this wouldn’t seem to change the situation for the better or for the worse, although the use of standards, embedded dictionaries, error-checking etc. within recording systems might appear to address some aspects of these problems.
However, Beck and Jones went on to argue that more problematic still is the use of published data used for comparative purposes:
“In such cases patterns observed are assumed to reflect archaeological patterns; it is rarely considered that there may be patterns that were created because of differences in analytic perception. In these cases a comparison of analyst consistency is unrealistic or impossible, and thus no fully conclusive test for analytical bias is available.” (1989, 245)
They conclude:
“The possibility for systematic analyst error exists in every artifact analysis and can have serious repercussions; it deserves the same careful attention as error introduced during data collection.” (1989, 260).
So it can be difficult to recognise bias in the data we collect – faults in our sampling processes, lack of consistency in our observations, differences between different observers, and so on. For example, Michael Given has identified an impressive array of factors which create or affect surface artefact densities. And it may be far more difficult to recognise bias in data which is somewhat removed from us, separated in time and space through the medium of publication. However, there are several ways the digital changes the situation:
- First, it can encourage the idea that we can do something about bias by applying technological solutions. This may be through changing our approach to collecting the data in the first place by introducing technological enhancements to the data capture process. This may be through technological controls over terminology and checks imposed on data, for instance, or more extensive modifications to the actual underlying methodologies. For example, Cesar González-Pérez (2012, 78) has proposed a form of typeless data modelling which he argues avoids category bias by bypassing classification as an a priori mechanism and uses the entity’s properties as the units of description. In the process, the focus is on what defines the entity, rather than what the entity is. However, the typeless approach tends to assume that properties are natural whereas their determination will in part be derived from personal experience, a priori knowledge, comparison with other objects, and hence still subject to bias. In answer, González-Pérez argues that the problem is reduced since properties are atomised relative to categories and do not determine the structure of the information in the way that categorisation does (2012, 84), but categorisation – and hence bias – may still be implicit, nonetheless. In addition, technologically-derived methods of data capture cannot be perceived as objective or unbiased since one or more subjective individuals lie behind the decisions about what is captured, how it is captured, what is significant, and what is considered not worthy or capable of being captured, as well as behind the design and implementation of the devices being used for capture purposes.
- Secondly, the digital can encourage the idea that we can address bias by virtue of the increasing availability and quantity of datasets online. We may seek to increase the size of our dataset and hence our analytical sample in an attempt to address the extent to which our data are representative of the original population from which they are drawn. However, simply increasing sample size can make the situation worse rather than better, as Clive Orton has pointed out (2000, 23), because it may disguise more significant underlying problems with the definition and characterisation of the data. It also entails incorporating data collected under different regimes, increasing the likelihood of inter-observer variation which is lost in the merging of different datasets. Imposing ontological structures on pre-existing datasets as a means of bringing them together runs this risk, and inter-observer bias is a fundamental problem which underlies most of our large online national archaeological databases, for instance.
- Thirdly, the digital increases the distance between analyst, data and the origins of those data. One of the key paradoxes that lies behind open data is that increasing access to increasing amounts of data has to be set against greater distance from that data and a growing disconnect between the data and knowledge about that data. As Faniel et al (2013) demonstrated, this does not stop archaeologists taking digital data out of context in their desire to reuse it, in the process setting aside their lack of knowledge about how the data were collected, what strategies were adopted, or – following Beck and Jones (1989, 260) – where the systematic analytical errors might lie. As currently employed, metadata does not address this situation, focused as it is on discovery rather than process. As Andrew Bevan has observed (2012, 493), access to online datasets brings with it problems associated with recovery and recording biases – and, I would suggest, a lot more besides.
Unconscious bias is a characteristic of digital archaeology. The digital facilitates an environment which can disguise, discourage or even deny closer investigation of biases surrounding the characterisation, categorisation, collection, cleaning, conversion, combination and processing of data within the hardware and software, tools and routines, black boxes and algorithms that we employ in our analyses and interpretations. And that’s before we consider the kinds of unconscious bias addressed by the course my employers are sending me on …
References
Beck, C. & Jones, G. 1989, ‘Bias and Archaeological Classification’, American Antiquity 54 (2), 244-262.
Bevan, A. 2012b ‘Spatial methods for analysing large-scale artefact inventories’, Antiquity 86, 492-506.
Faniel, I., Kansa, E., Witcher Kansa, S., Barrera-Gomez, J. & Yakel, E. 2013 ‘The challenges of digging data: a study of context in archaeological data reuse’, Proceedings of the 13th ACM/IEEE-CS Joint conference on Digital libraries (JCDL ’13), ACM New York, pp 295-304. http://doi.acm.org/10.1145/2467696.2467712
González-Pérez, C. 2012 ‘Typeless information modelling to avoid category bias in archaeological descriptions’, in A. Chrysanthi, P. Murrieta Flores & C. Papadopoulos (eds.) Thinking beyond the Tool: Archaeological computing and the interpretive process (BAR International Series 2344, Archaeopress), 72-87.
Orton, C. 2000 Sampling in Archaeology (Cambridge University Press)
Perry, S. 2014 ‘Cyber-abuse of academics – it’s time for action‘, Times Higher Education, 13 February 2014: 30.
Perry, S., Shipley, S. & Osbourne, J. 2015 ‘Digital Media, Power and (In)Equality in Archaeology and Heritage‘, Internet Archaeology 38.